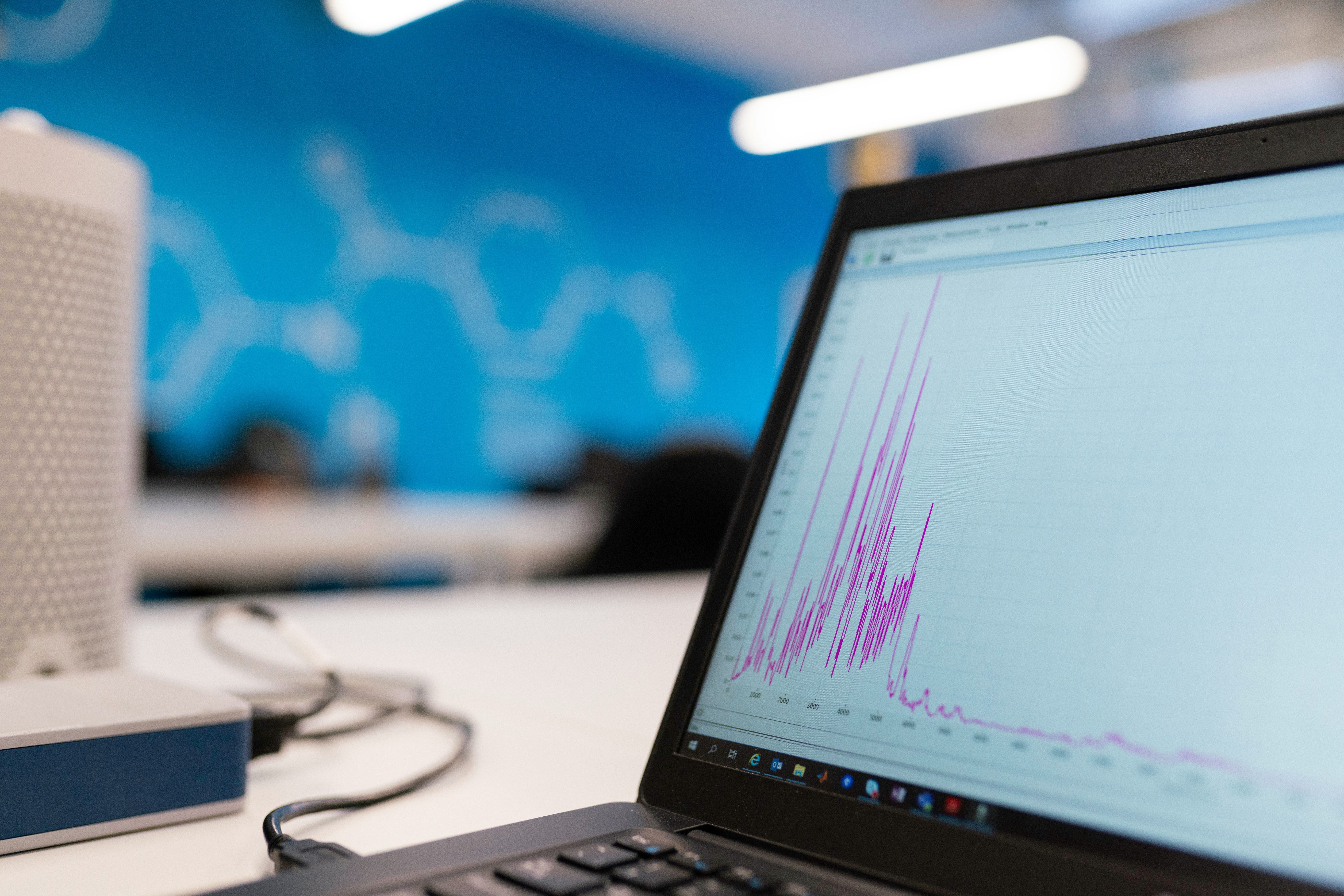
Clinical research is the cornerstone of modern medical science, providing the foundation for innovative treatments, therapies, and drugs. At the heart of this research lies statistical analysis, which plays an indispensable role in shaping the outcomes of clinical studies. Statistical analysis empowers researchers to interpret complex data, draw reliable conclusions, and make informed decisions. As clinical research continues to evolve, the importance of statistical techniques becomes increasingly evident, driving both the pace and precision of medical advancements.
Enhancing Data Interpretation
Statistical analysis is vital for managing and interpreting the vast amounts of data generated in clinical trials. Clinical studies often involve large sample sizes, which produce intricate datasets that can be overwhelming without the right analytical tools. Researchers can identify patterns and trends that may not be immediately apparent by applying statistical methods. For instance, statistical tests can highlight significant differences between treatment groups, allowing researchers to determine whether the observed effects are due to the treatment or random occurrences.
Beyond identifying trends, statistical analysis also helps manage the variability inherent in clinical research. In clinical trials, participants come from diverse backgrounds, each with unique genetic, environmental, and lifestyle factors that could influence their response to treatment. Statistical methods like regression analysis help researchers account for these variables, ensuring the results are as accurate and generalizable as possible. By controlling for such factors, researchers can ensure that their findings reflect the impact of the studied intervention.
Validating Hypotheses and Predictions
One of the most significant roles of statistical analysis in clinical research is its ability to test hypotheses and make predictions. Before embarking on a clinical trial, researchers formulate hypotheses based on existing knowledge or preliminary studies. These hypotheses outline what researchers expect to find and guide the study’s design. Statistical analysis is then used to test these hypotheses by analyzing the collected data and determining whether the results support or refute the initial predictions.
By conducting hypothesis testing, researchers can ensure that their conclusions are not merely coincidental but are supported by statistical evidence. This rigorous approach increases the credibility and reliability of clinical research, especially regarding regulatory approval of new treatments or drugs. Furthermore, statistical techniques such as predictive modeling enable researchers to forecast potential outcomes based on existing data. This ability to predict patient responses, treatment effects, and long-term consequences can significantly improve the efficiency of clinical trials, allowing researchers to make necessary adjustments in real time.
Minimizing Bias and Ensuring Precision
Clinical research is susceptible to various biases, such as selection bias, measurement bias, or other forms of systematic errors. Statistical analysis safeguards against these biases by employing techniques that reduce their impact on the study’s results. Randomized controlled trials (RCTs), which are considered the gold standard in clinical research, heavily rely on statistical methods to minimize bias. By randomizing participants into different treatment groups, researchers ensure that the groups are comparable, reducing the likelihood of confounding factors influencing the results.
Moreover, statistical analysis ensures precision by calculating confidence intervals and p-values, which offer insights into the reliability of the study’s findings. Confidence intervals provide a range of values within which the actual effect is likely to fall, while p-values help determine the statistical significance of the results. Together, these statistical tools help researchers gauge the strength of their findings and whether the observed effects are likely to be reproducible in broader populations. With this level of precision, clinical research can produce more accurate and dependable results, ultimately benefiting patients and healthcare systems.
Optimizing Clinical Trial Design
The design of a clinical trial plays a crucial role in the reliability of its results. Statistical analysis is integral to designing trials that are both efficient and effective. For example, sample size calculation is a key element of trial design, and statisticians use power analysis to determine the optimal number of participants required to detect meaningful differences between groups. A trial that is too small may fail to identify significant effects, while one that is too large may waste resources and expose more patients to potential risks than necessary.
In addition to determining sample sizes, statistical analysis helps select appropriate endpoints and metrics to measure a trial’s success. For instance, when assessing the effectiveness of a new drug, researchers must choose the proper clinical outcomes, such as survival rates, symptom reduction, or quality of life, that accurately reflect the drug’s impact. Statistical methods allow researchers to define these endpoints clearly, ensuring the data collected will be meaningful and aligned with the trial’s objectives.
Improving Post-Trial Analysis and Follow-up
Even after a clinical trial, statistical analysis is vital in the post-trial phase. Once the data is collected, it needs to be thoroughly analyzed to understand the full implications of the findings. Statistical techniques help researchers perform sensitivity analyses, which test the robustness of the results under different assumptions or scenarios. This step is essential for ensuring that the conclusions drawn from the data are valid and applicable to real-world situations.
Moreover, statistical methods are crucial for conducting long-term follow-up studies that assess the enduring effects of a treatment. Many interventions require further monitoring after a clinical trial ends to understand their long-term safety and effectiveness. Statistical analysis enables researchers to track patient outcomes over extended periods, providing valuable insights into the’ long-term benefits or risks of treatments. By employing sophisticated statistical models, researchers can examine how factors, such as age, comorbidities, or treatment adherence, affect long-term outcomes.
In clinical research, statistical analysis is not merely a tool for crunching numbers; it is an essential component of the research process that drives scientific discovery and patient care. From enhancing data interpretation to minimizing bias and optimizing trial design, statistical analysis ensures that clinical research yields valid, reliable, and actionable results. As clinical trials become increasingly complex and involve more intricate data, the importance of statistical methods will continue to grow. Ultimately, integrating statistical analysis into clinical research accelerates the development of new treatments, improving health outcomes and advancing medical science.